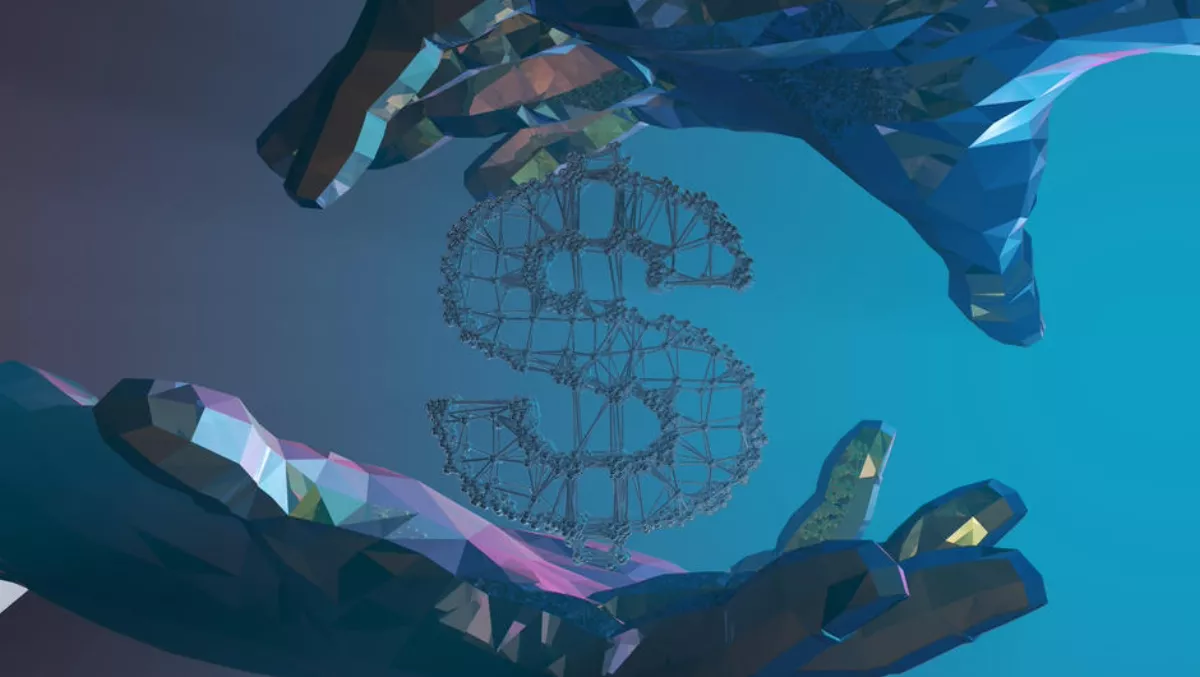
GTC18 - SAS on how to make money with deep learning
SAS chief data scientist Wayne Thompson wants people to know that implementing and getting a return on investment (RoI) with deep learning is within reach for almost any business.
Thompson's session, 'The Path to RoI with AI', begins with him explaining how there are plans for drones and deep learning to enhance conservationists' tracking capabilities and improve understanding of migration and behavioural patterns (he also encourages people to donate to WildTracks, a charity for the conservation of endangered species.)
"AI is hot - it's roughly a (US)$40 billion business by 2020," Thompson says.
"It is not just for Google, Facebook... AI is for everybody and I think you should start talking about yourselves like Capital One does - as a software company - like Amazon does.
The reasoning is fairly straightforward - Whatever it is that your company does, AI can help you leverage your data to make money.
"Who ended up buying Borders' online business? Amazon," he adds to highlight his point.
To show the diversity of the value AI has to offer, Thompson highlights a few more cases where AI applications have helped improve revenue.
One example is SciSports, a Dutch company that is using cameras in a football (soccer) stadium backed by a neural network on an NVIDIA GPU that can tell who a player is, identify their jersey number and follow the ball, and then report that data in order to rank players.
"We only had 10,000 images to start with. This is the big problem with deep learning - that is not a lot of images - but that's what we did with them as a development partner," Thompson says.
"We built this software with this customer and I recommend that to anyone out there - If you are trying to build a data product do it with someone who has data and an application for it.
Backed by all of his experience in applying AI and deep learning in specific use-cases, Thompson offers advice on how to get going on AI in a way that will lead to RoI.
"First of all, you've got to get a lot of data, and you've got to get data to solve one particular problem," he says.
"Then, most importantly, you start to get users working with that data. You then get more data from the users and so it becomes kind of a circle. You start that machine, like a deep learning model, and you just keep pressing it.
"You take one problem, get the data around it, make sure you get people working with you and using it up front, and then you start to get more data. That's how I build product with SAS and that's how I encourage my customers to build.