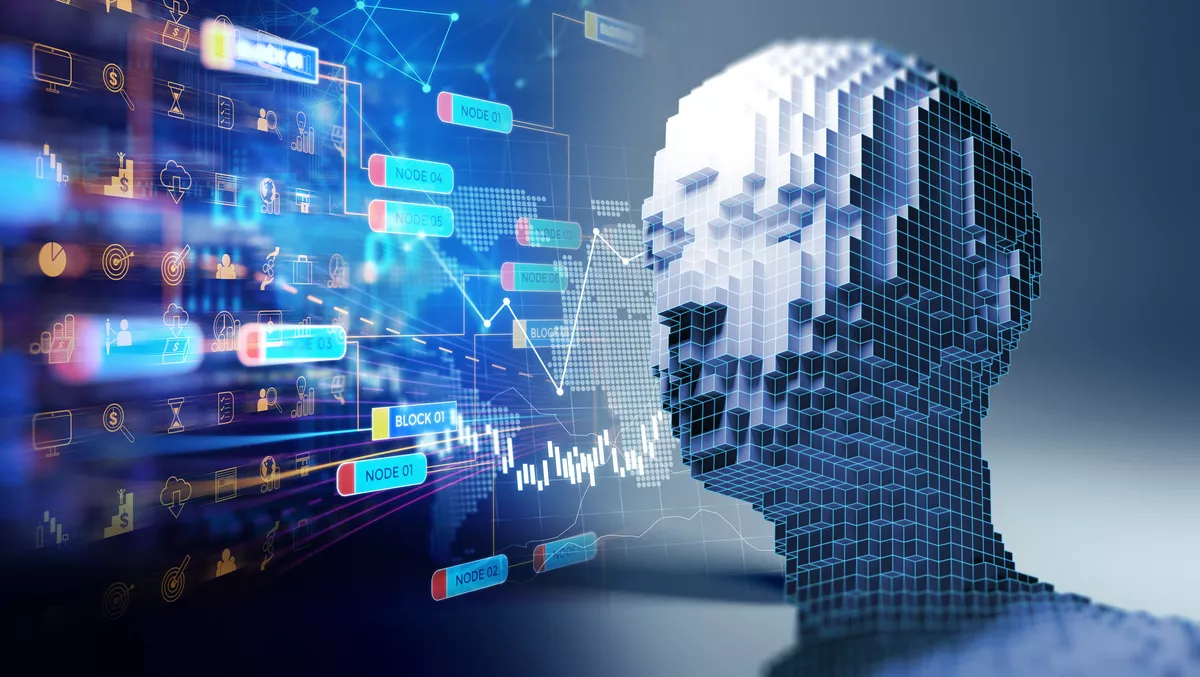
H2O.ai democratises deep learning with H2O Hydrogen Torch
H2O.ai has announced H2O Hydrogen Torch, a deep learning training engine that makes it easy to make state-of-the-art image, video and natural language processing (NLP) models without coding.
The company says that creating deep learning models has required extensive data science knowledge and time until now. "H2O Hydrogen Torch was developed by the world's best data scientists, Kaggle Grandmasters, and the challenging parts of creating world-class deep learning models are handled automatically by the product," the company says.
Through a no-code user interface, data scientists and developers can rapidly make models for multiple image, video and NLP processing use cases, including identifying or classifying objects, analysing sentiment or finding relevant information in text.
"According to multiple analyst estimates, 80% to 90% of data is unstructured information, yet only a small percentage of organisations can derive value from unstructured data," says H2O.ai CEO and founder, Sri Ambati.
"Deep learning models provide the ability to unlock opportunities to transform industries including healthcare, including computer-aided disease detection or diagnosis through the analysis of medical images. Insurance, in areas such as automation of claims and damage analysis from reports and image, and manufacturing in predictive maintenance by analysing images, video and other sensor data."
One use case is Aura.ceo is a talent screening platform that offers a data-driven, outside-in perspective on an organisation's workforce. By using public data from a range of sources, Aura. ceo's interactive platform enables its customers to evaluate the array of roles, skills and experience inside a company of any size and see how it compares to competitors.
"H2O Hydrogen Torch has been a key enabler in helping us operationalise machine learning for shifting data," says Aura.ceo CTO, Stelios Anagnostopoulos. "We can get from a new dataset to a deployed model and updated tables in our data warehouse in a couple of days instead of weeks."
Image and video processing
H2O Hydrogen Torch can be trained for classification, regression, object detection, semantic segmentation, and metric learning for images and videos. For example, in a medical setting, H2O Hydrogen Torch could analyse medical X-ray images for abnormalities with a 'human in the loop' to make the final decision. Other image-based use cases include object detection in a manufacturing facility to determine whether a part is missing, or metric learning that alerts an online retailer to duplicate images on a website.
Natural language processing
For text-based or NLP use cases, H2O Hydrogen Torch can be trained for text classification and regression, token classification, span prediction, sequence-to-sequence analysis and metric learning.
These use cases include predicting customer satisfaction from transcribed phone calls to sequence-to-sequence analysis to summarise a large portion of text, such as medical transcripts, in a few sentences.
These models then can be packaged automatically for deployment to external Python environments or in a consumable format directly to H2O MLOps for production.
"Accelerated by COVID-19, video streams, speech, audio podcasts, email, and natural language text have become the fastest-growing data for our customers in every industry," says Ambati.
"Transforming and fine-tuning pre-built deep learning models to deliver high accuracy requires a no-code AI Engine to democratise AI for these use cases. H2O Hydrogen Torch does exactly that by bringing best practices from Grandmasters to tackle problems ranging from improving in-store customer experiences, identifying fashion trends, and discovering vaccines to saving lives with video-enabled drones fighting fires with AI on the edge.
"With H2O Hydrogen Torch as a core AI Engine of the H2O AI Cloud, our customers can train models in deep learning and better serve their customers and challenge tech giants," he says.