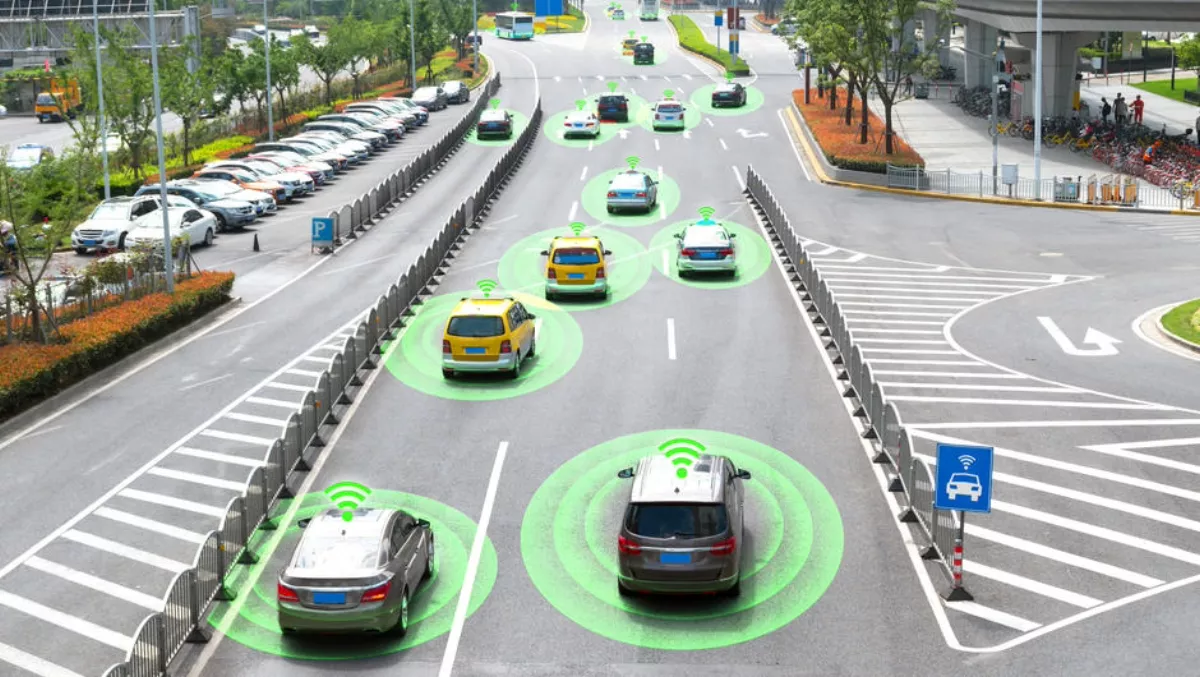
What we can learn from the car’s journey to AI
Embedded design since 1981
The first car was sold in 1885 - this was the beginning of a revolution through motorised means of transport and a revolution for society. Vehicles have become faster, more aerodynamic, safer and more comfortable over time.
They established themselves as a means of mass transportation and brought people unprecedented mobility. Initially, however, all progress in vehicles was related purely to the engineering level.
It was not until almost 100 years later, in 1981, that the first computer was installed in a vehicle. A General Motors model had an ECU with about 50,000 lines of code. The engineers of that time could not imagine the wealth of new possibilities they created as a result.
Nevertheless, the model, which is the pioneer of embedded design, so to speak, represents the initial spark for all further developments: Battery management, airbags, electric windows or automatic locks. Today, more than 100 processors with around 100 million lines of code are installed in every car.
From the first computer in the vehicle to the connected car
Another milestone in the software development of the automobile is the possibility of networking. The first Connected Car was launched in 1996. At first, it only offered a mobile phone to contact a support centre. But it could quickly send GPS coordinates or even information on the condition of the vehicle. Shortly thereafter, in 2000, the first driver assistance system followed: a warning system when a Mercedes truck accidentally crosses the lane.
Today, automobiles have finely tuned predictive maintenance functions that send warnings before a particular component stops working. There are functions that can be controlled by voice to keep both hands on the wheel. Vehicle-to-Vehicle applications pass on important information from one vehicle to another, by electronically forewarning other cars of emergency braking or changing lanes around blind spots. And in the next few years, manufacturers will launch the first autonomous cars on the market.
The second digital revolution
What we see from these examples is the second digital revolution in the automotive industry, which is all about bringing artificial intelligence into the vehicle. It is supported by Model-Based Design. One example is the emergency braking system developed by Scania for their trucks to avoid rear-end collisions. In this case, radar and camera sensors are used in combination to detect the environment.
In particular, radar sensors can determine the range of an object, its relative speed and strength - in other words, detect whether it is a fog bank or a solid object. Cameras add further important information to this picture. You can determine the size of an object and its lateral position to determine whether the vehicle is in its own lane or parked on the hard shoulder. As soon as a collision is imminent, the driver is warned by an acoustic signal. If this does not react, a warning brake is automatically applied.
How the vehicle becomes intelligent
In a system like Scania's, a large amount of new data is evaluated every second and needs to be analysed in real time: The information from radar sensors and cameras must be evaluated and merged into a uniform image. Then we have a decision to make: Is there any danger? Does emergency braking need to be prepared? Any other points to bear in mind during development? Is the code that does these calculations efficient? At Scania, CPU utilisation of the electronic control unit was already 60% when the company started the sensor function project.
To ensure that the emergency braking mechanism works reliably, it is thoroughly tested during development. Scania verified its design using simulations based on more than 1.5 million kilometres of sensor data using model-based development.
Machine learning for engineers
The development of increasingly complex applications and vehicle functions presents engineers with a major challenge: How can the huge amounts of data supplied by the sensors be efficiently evaluated? How do you find the data that really make a difference? How can they be used to provide customers with better service?
One solution can only be to combine the expertise of automotive engineers with the know-how of machine learning techniques and data analysis. Several companies today are helping to provide automotive engineers with the tools they can use to analyse big data and build machine learning applications themselves.
Automotive companies are increasingly relying on extending their extensive knowledge of tools like MATLAB to include AI development, which reduces the learning curve required with other deep learning tools. With over three decades of experience offering automotive companies design and development support, MATLAB also offers immediate access to prebuilt functions, extensive toolboxes, and specialised apps for classification, regression, and clustering.
Over the last few years, the automotive industry has seen a digital revolution, with the addition of electronic controls in virtually every system. Now, automated driving and predictive maintenance are ushering in the next phase of evolution, in which data-driven algorithms for implementing artificial intelligence are playing a key role. The winners will be determined by their ability to merge automotive engineering with big engineering data, making analytics and deep learning part of their research, design and development processes.