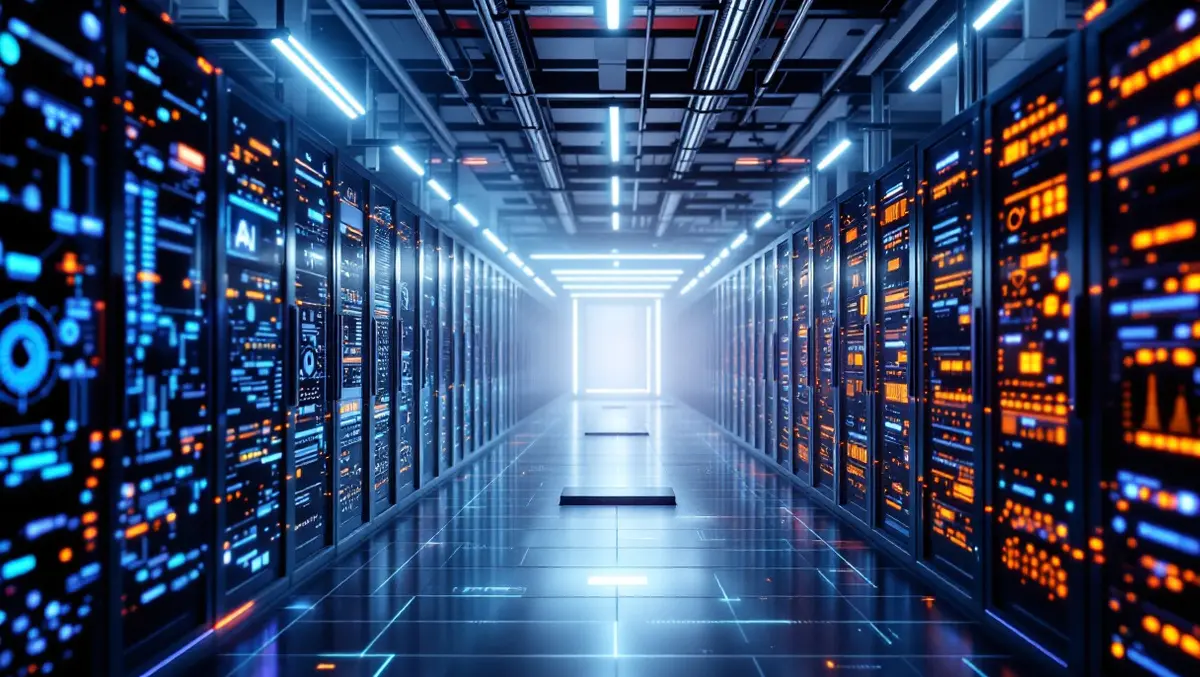
Google Cloud unveils stronger AI supercomputing infrastructure
Google Cloud has announced advancements in AI supercomputing infrastructure through its AI Hypercomputer, introducing hardware and software upgrades designed to deliver improved intelligence per dollar for AI workloads.
The latest release, known as Ironwood, represents Google Cloud's 7th generation TPU focused on inference, featuring a peak compute capacity five times greater and a sixfold increase in high-bandwidth memory compared to the previous Trillium generation. Google Cloud describes Ironwood as 2x more power-efficient and available in two configurations, each providing an efficient compute offering for developers via its optimized stack available across PyTorch and JAX.
In terms of virtual machines, A4 VMs, utilising NVIDIA B200, have been available since a recent NVIDIA event, while A4X VMs, using NVIDIA GB200, are now entering preview stages. The company also introduced enhanced networking capabilities with its new 400G Cloud Interconnect for improved bandwidth, allowing for better connectivity across environments. Moreover, Hyperdisk Exapools provide a high-performance block storage capacity for AI clusters, enabling significant storage capabilities and throughput with exabyte scale storage potential.
In relation to storage enhancements, Google Cloud debuted Rapid Storage and Cloud Storage Anywhere Cache. Rapid Storage allows co-location of primary storage with TPUs or GPUs for optimal utilization, while Anywhere Cache offers a consistent read cache to reduce latency by 70%, maintaining data proximity to accelerators.
On the software front, Google's AI Hypercomputer stack supports various machine learning frameworks, including PyTorch, JAX, vLLM, and Keras. The Pathways system, developed by Google DeepMind, is now available, enabling scalable training and inference with dynamic processes for ultra-low latency and throughput optimised workloads.
New features for training workloads focus on cluster management through Cluster Director for GKE and the forthcoming Cluster Director for Slurm, designed to support GPU and TPU processing. The integrated features provide dashboards for cluster utilisation, AI Health Predictor and Straggler Detection, along with job continuity features that ensure training continues uninterrupted during node failures.
In addressing the demands of AI inference workloads, AI inference capabilities are being introduced within Google Kubernetes Engine (GKE), offering cost and performance improvements. GKE Inference Gateway provides intelligent scaling and load-balancing, while GKE Inference Recommendations assist in matching infrastructure needs with the right model performance goals.
The release also highlighted vLLM's support for TPUs, enabling fast and efficient library inference operations across various Google services, including Compute Engine and Vertex AI. Additionally, the Dynamic Workload Scheduler (DWS) has expanded, and now accommodates new accelerators, allowing for flexible resource provisioning suitable for both inference and training workloads.
Mark Lohmeyer, Vice President of Compute and AI Infrastructure, remarked, "Today's innovation isn't born in a lab or at a drafting board; it's built on the bedrock of AI infrastructure." George Elissaios, Vice President of Product Management for Compute Engine and AI Infrastructure, added, "AI Hypercomputer is an integrated supercomputing system that's distilled from more than a decade of Google's expertise in AI."
The developments from Google Cloud aim to facilitate AI workloads' demands with a harmonious blend of hardware and software, addressing efficiency and cost considerations for users in the evolving AI landscape.